ABSTRACT
The standardized mean difference (SMD) is the difference between the means of a variable, expressed not in its original unit but in the unit of standard deviation (SD). SMDs of 0.2, 0.5, and 0.8 are conventionally considered to be small, medium, and large, respectively. The reader, however, obtains no real world understanding of an SMD from these adjectives. This article suggests a solution: SMDs and their 95% confidence intervals can be better understood if they are converted into percentile scores. The procedure is explained, step by step, with reference to a meta-analysis that found that cholinesterase inhibitors (ChEIs) significantly attenuated delusions and hallucinations in Alzheimer disease and Parkinson disease with SMDs that ranged from −0.08 to −0.14. After conversion of these SMDs to percentile scores, the reader is shown that the average patient in the ChEI treatment arms would have improved by just 3 to 7 percentile places relative to the average patient in the placebo arms. So, whereas the findings were statistically significant, they would perhaps be so small as to be clinically unobservable in the average patient. All that the reader needs to do to convert an SMD into a percentile score is to locate a table that presents area under the normal curve, understand how the table presents what it does, look up the SMD value in the table, and obtain the percentile score from the value in the table. The entire procedure is very easily understood and takes less than a minute, starting from locating the table through an online search to obtaining the percentile score for the SMD.
J Clin Psychiatry 2023;84(4):23f15028
Author affiliations are listed at the end of this article.
Research is conducted with prespecified objectives. These objectives may be operationalized into hypotheses. A hypothesis is a statement of an expected relationship between variables. This relationship between variables is examined in statistical analyses. The results of statistical analyses are presented as numbers. These numbers describe aspects of the relationships that were examined. The numbers are usually accompanied by confidence intervals and, where appropriate, by P values that declare whether statistical significance is met or not.
The numbers that emerge from analysis are called statistics; statistics describe the sample. Examples of statistics include incidences and prevalences, means and standard deviations (SDs), response and remission rates, and numbers needed to treat or harm. Examples also include correlations, mean differences, standardized mean differences (SMDs), relative risks, odds ratios, hazard ratios, and others. Most of these statistics are easy to understand. Some, however, are more difficult to get a grip on; the SMD is one such statistic.
Standardized Mean Difference
The SMD was explained in an earlier article in this column.1 In summary, the SMD is the difference between the means of a variable, obtained from 2 independent groups (or from the same group at 2 different points in time), expressed not in its original unit but in the unit of SD. There are 2 common contexts in which the SMD is used. One is as a measure of effect size in a single study, especially when the outcome is measured with an instrument with which the reader is unfamiliar. The other is as a measure of effect size in meta-analysis, especially when outcomes in different studies are measured using different instruments. This is explained in greater detail in Box 1.
Interpretation of the Standardized Mean Difference
The SMD is a number. It stands alone; the unit, which is the SD, is implicit; it is not stated. How may one interpret this number?
By convention, SMDs of 0.2, 0.5, and 0.8, regardless of (positive or negative) sign, are considered to be small, medium, and large, respectively.1,2 So, as an example, if we find in our study that an outcome improved significantly in Group 1 relative to Group 2, and that the SMD was 0.18, we would conclude that the outcome was better in Group 1 by “only” 0.18 SDs; that is, the effect size was small.
As a digression, here, relative risks, odds ratios, hazard ratios, numbers needed to treat or harm, and so on are all measures of effect size.2 However, when people use the phrase “effect size,” chances are that they are referring to the SMD.
As another digression, the SMD may be estimated in different ways. Examples are Cohen d, Hedges’ g, and Glass’ delta.1,2 Regardless of the method of estimation, the method of interpretation of the value is the same.
Now, here is a problem. Saying that an SMD of 0.2 is small merely uses an adjective in place of a number; it gives us no everyday understanding, whatsoever, of the smallness. Likewise, saying that an SMD of 0.8 is large does not give us a feel of the largeness. Units such as kilograms on the weighing scale at our feet and centimeters on a tape around the waist are easily understood because of an everyday familiarity with the units. Can the SMD also be translated into everyday terms? The answer is yes, if one converts the SMD into a percentile. This is a simple trick that takes a little effort to describe but is easily understood and easily performed.
Percentiles
Most readers will be familiar with the concept of percentiles. In short, an adult Caucasian male who is at the 70th percentile in height is taller than 70% of the population of adult Caucasian males and shorter than the remaining 30%; a 10-year-old child who scores above the age-standardized 97th percentile on a test lies in the top 3% of the population of 10-year-olds with regard to performance on that test.
Here are some notes about the percentile. The percentile gives us no information about the actual height or actual score on the test. The percentile is a measure of ranking; in contrast, a percentage is an actual score (for a continuous variable) or a proportion (for a categorical variable) that has been standardized for a range of 0 to 100. The 50th percentile is the median value in the population: half of the population lies below and half lies above. Finally, percentiles can be obtained for both samples and population.
Using the Standard Deviation to Locate a Percentile
There is a definite and well-known relationship between the mean and the SD in the normal distribution.3 So, if we have a single value, and we know how far above or below the mean this value lies when it is expressed in units of SD, we can pinpoint the position of the value in the normal distribution. When we do this, we will know how much of the normal distribution lies below vs above the value; that is, we get a percentile score.
As a worked example, assume that we have a test that has a mean value of 100 and an SD of 15. Then, a person with a score of 115 will lie 1 SD above the mean. But, from the properties of the normal distribution, we know that the mean ± 1 SD includes 68.26% of the population. So, the mean + 1 SD will include 34.13% of the population. Now, the mean in a normal distribution coincides with the median; that is, it lies at the 50th percentile mark. So, the mean + 1 SD will lie at the 50.00 + 34.13 or the 84.13 percentile mark. That is, a person with a score of 115 is at approximately the 84th percentile.
The SMD is expressed in units of SD. That is, depending on its sign (positive or negative), it tells us how many SDs above or below the mean our outcome of interest lies. So, just as in the worked example above, we can convert an SMD into a percentile score.2 We can now conclude that an SMD of 1.00 lies at the 84.13 percentile mark. There is a very easy way to go about doing this for other values of the SMD, as explained in a later section.
Application to an Actual Study
In a systematic review and meta-analysis of randomized controlled trials, d’Angremont et al4 found that cholinesterase inhibitors significantly reduced ratings of delusions and hallucinations in patients with Alzheimer disease and Parkinson disease. The SMDs lay in the −0.08 to −0.14 range.
We can straightaway draw 2 conclusions. One is that, because the SMDs are negative in sign, treatment was associated with reduction in ratings of delusions and hallucinations; that is, in clinical improvement. The other is that, because the values 0.08 and 0.14 are less than 0.2, the magnitude of the improvement was “small.”
How small was it? This can be determined by converting the SMDs into percentile scores, as described in the next section.
Step-by-Step Instructions
Find a table that presents the area under the normal curve. Such a table can be identified online, within seconds, using the search phrase (without quotes) “table for area under the normal curve.” The table will be discovered either directly, with this search, or when the “images” results for the search are examined. Such a table can also be found among the appendices of textbooks on statistics and research methodology, or among the appendices of other textbooks, as well, including textbooks of psychology. The table may be titled “area under the normal curve” or “area under the normal distribution” or “area under the cumulative normal distribution,” or “z score table” or otherwise.
There are at least 3 different ways in which information may be presented:
Scenario 1. The z value 1.00 (1 SD or z = 1.00) corresponds to the number 0.8413. This straightaway tells us what we had ourselves worked out in a previous section: that SMD = 1 corresponds to the 84.13 percentile mark. This is the easiest table to work with, and readers are recommended to identify and use this table.
Scenario 2. The z value −1.00 corresponds to 0.1587. This also tells us what we want to know: that, because 15.87 lies on the “lower” side, SMD = 1 corresponds to the 84.13 percentile mark (note that 15.87 + 84.13 = 100.00, which is the total area under the normal curve).
Scenario 3. The z value 1.00 corresponds to 34.13. This also corresponds to what we had worked ourselves worked out in a previous section: that SMD = 1 corresponds to mean + 1 SD, or 50.00 + 34.13, or the 84.13 percentile mark.
Putting the Instructions to Practice
In the d’Angremont et al4 study, the statistically significant SMDs lay in the −0.08 to −0.14 range. The minus sign can be ignored as long as it is remembered that the SMDs in this meta-analysis indicate improvement. Let us now apply our newly learned knowledge to the conversion of these SMDs into percentiles.
Using the table described in Scenario 1, above, note that the z value 0.00 corresponds to 0.5000, that is, the center of the normal distribution, or the 50th percentile. Now, look up z = 0.08. You will find that it corresponds to 0.5319, or the 53.19 percentile mark. An SMD of 0.08 means that if the average patient in the placebo group is at the 50th percentile, the average patient in the cholinesterase inhibitor group will move up by just 3.19 percentile places. This is a very small improvement in real terms, even if it is statistically significant. You will obtain the same result, though by a different route, should you use the tables described in Scenarios 2 and 3 above.
It can likewise be determined that an SMD of 0.14 moves the average patient up by 5.57 percentile places; that is, from 50.00 to 55.57. Again, this is a very small improvement in real terms, even if it is statistically significant. We now understand why the statistically significant findings in the meta-analysis by d’Angremont et al4 are unlikely to be clinically significant.
This method can also be used to find the percentile positions for the 95% confidence interval values around the SMD.
Readers may note that although this explanation was long, it is easy to understand and can be applied to an SMD in only a few seconds provided that the appropriate table is available (and this table can be found in seconds through an online search). So, the time and effort involved are small.
Other Notes
Using the method described in this article, it is seen that an SMD of 0.2 corresponds to an improvement of 7.93 percentile places. The reader will now understand why an SMD of 0.2 is considered “small.”
SMDs in neuropsychiatric research are commonly small to medium in range. It is not usual to obtain large SMDs, that is, SMDs that are greater than 0.8.
In longitudinal research designs, when a treatment effect is being examined, the SMD is usually larger when outcomes are compared before and after intervention in the experimental group than when they are compared after intervention between the experimental and control groups. This is because in a post- vs pre-treatment contrast, placebo mechanisms enhance the SMD, but in experimental vs control group contrasts, the placebo effects tend to cancel out. So, readers should not be carried away by SMDs that may be > 1 in single-arm analyses or in unblinded studies from which placebo effects cannot be adjusted for.
The values 0.2, 0.5, and 0.8 that indicate small, medium, and large effect sizes are suggestions; they are not set in stone.2 The SMD should therefore be interpreted in context. For example, for outcomes that have major health implications, even a small value for SMD may be clinically worthwhile, and especially so from a population or public health perspective.
Article Information
Published Online: August 7, 2023. https://doi.org/10.4088/JCP.23f15028
© 2023 Physicians Postgraduate Press, Inc.
To Cite: Andrade C. How to use percentiles to better understand standardized mean difference (SMD) as a measure of effect size. J Clin Psychiatry. 2023;84(4):23f15028.
Author Affiliations: Department of Clinical Psychopharmacology and Neurotoxicology, National Institute of Mental Health and Neurosciences, Bangalore, India ([email protected]).
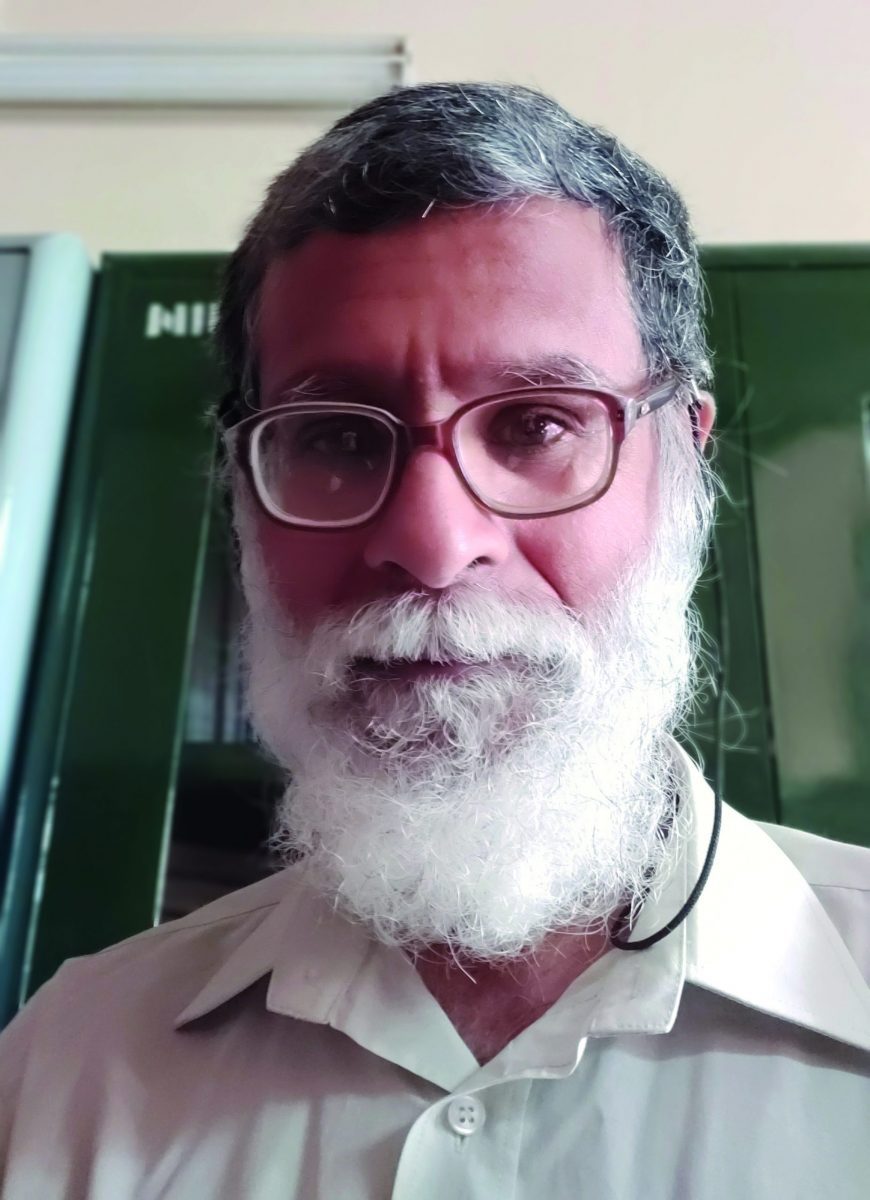
Department of Clinical Psychopharmacology and Neurotoxicology, National Institute of Mental Health and Neurosciences, Bangalore, India ([email protected]).
Financial disclosure and more about Dr Andrade.
References (4)
- Andrade C. Mean difference, standardized mean difference (SMD), and their use in meta-analysis: as simple as it gets. J Clin Psychiatry. 2020;81(5):20f13681. PubMed CrossRef
- Durlak JA. How to select, calculate, and interpret effect sizes. J Pediatr Psychol. 2009;34(9):917–928. PubMed CrossRef
- Norman GR, Streiner D. Biostatistics: The Bare Essentials. 4th ed. Shelton, Connecticut: People’s Medical Publishing House; 2014.
- d’Angremont E, Begemann MJH, van Laar T, et al. Cholinesterase inhibitors for treatment of psychotic symptoms in Alzheimer disease and Parkinson disease: a meta-analysis. JAMA Neurol. 2023;26:e231835. PubMed CrossRef
This PDF is free for all visitors!